Professor Genevieve Bell
Distinguished Professor, Florence Violet McKenzie Chair Director, Autonomy, Agency & Assurance, Australian National University
‘Well, so the good news is you were primed for divergent thinking. The bad news is I’m your divergent thinker. So listen, it is my incredible pleasure and privilege to get to be here. Unlike the previous professor I find this a slightly more daunting experience. There’s no nostalgia for me being in a room full of uniforms, mostly just a bit of fear; I fear it may remind me of school and that’s not always good.
‘I thought I’d take this opportunity to move the conversation in a slightly different direction. So you’ve heard an explication of what it means to think about emerging and future technologies in the context of land warfare. You’ve heard an incredible explication of what that means around the region we find ourselves in. I want to move the conversation in a slightly different direction and talk explicitly about one of the pieces of technology that I think is going to be critical in this space. And I really want to auger in on this notion of artificial intelligence: how we might define it, how we should think about it, and what the consequences are.
‘I realise before I do that you might need just a little bit of background on me because I don’t read as a traditional person inside this conversation. I am indeed Australian; born in Sydney, raised all over the place. I spent most of my childhood in central and northern Australia in the 1970s and 1980s. My mother is also a cultural anthropologist and I grew up on her field sites. So I grew up with indigenous people in a time when people were still closer in some ways to their country. So I grew up in a time when I didn’t speak English—I spoke Warlpiri. I spent most of my time out of school and I went hunting and gathering with people every day who told me stories about their country. Oh and I got to kill things; and of course eat them, let’s be clear. It was a remarkable childhood and it’s one that means that whenever I get to come to events like this, the welcome to country means something very special to me. So it was lovely to see Kath [Major General Kathryn Toohey – Ed.] acknowledge that we’re meeting on Kaurna country and that we are on the land of the Kaurna and the Ngarrindjeri and the Ramindjeri people.
For me as an Australian having spent 30 years abroad, one of the loveliest things about coming home is that I get to acknowledge that I’m always on a country that’s been occupied for 60 000 to 100 000 thousand years and that whenever we have a conversation about the future in Australia, we are having that against the backdrop of the longest continued human settlement on the planet. And so what it means to acknowledge that we stand in that place and with those people is not just acknowledging the elders past, present and future but acknowledging both our history and our responsibility to that history. So for me getting to say that always means something special. Because—30 years in America— no offence, [to Americans in the audience – Ed.] you guys never acknowledge it, and you also have indigenous people who have those same histories, so for me getting to talk about that [it’s] kind of powerful.
You can imagine though it’s a really long way from being a barefooted kid who speaks an Aboriginal language to Silicon Valley. And I did it the usual way. Like all good Australians, I ran away from home. I went to America, I got myself into a decent American university. I got myself into an even better American university and I found myself in Silicon Valley in the 1990s. My doctoral work was actually a history of one of the first boarding schools for Native American kids. Some of you will know that school because it is now the Army War College in Carlisle, Pennsylvania. But between 1879 and 1918 it was the Carlisle Indian School and it took 10,000 Native American kids from 140 different nations and created an educational experiment the likes of which has never been seen since. For those of you who are Americans in the room you’ll also know it because of course, Pop Warner was the football coach and Jim Warner was his star student.
‘How I ended up at Intel is a mystery to almost everyone, except that it all starts with a man in a bar. No, I’m serious. I got my job at Intel because I met a man in a bar. In Palo Alto in 1998 when I’d finished my PhD and I was on the faculty at Stanford, I met a man in a bar who asked me what I did. I told him I was an anthropologist. He said: “What’s that?” I said I studied people for a living. He asked: “Why?” I should have known at that point he was an engineer.
I just didn’t know better. He asked me what I did with that. I said I was a professor. He said couldn’t I do more and I thought: “Yes. I could stop speaking to you”, which indeed I did.
‘So you could imagine my surprise when he called me at my house the next day. Because we’re talking 1998, before LinkedIn, before Facebook, before Twitter, before Tinder, even before Google. So we’re talking before the white box on the internet, and in fact he found me the old-fashioned way: he called every anthropology department in the Bay Area and asked for a redheaded Australian. Stanford’s anthropology department said: “Do you mean Genevieve? Would you like her home phone number?”
‘So basically, I got my job at Intel because of bad security practices and men in bars and I’ve spent 20 years at Intel. It is actually my 20th anniversary this week and my job there was always to think about what is the relationship between people and the process of making new technology. So how do we not just do the work that is technically possible but how do we make technology that people care about; that solves people’s problems; that addresses people’s fundamental needs. And the way I’ve always thought you do that is that you actually have to go and spend time with people and the places they make meaning in their lives and understand what makes them tick. And so at Intel we used those kinds of insights to drive new product development. Thinking about what was technically possible was important; thinking about what would work at a human scale was equally important.
‘I’ve been doing that job for 20 years. I came home to Australia two years ago specifically to start thinking about how all of that might apply to artificial intelligence. Part of the reason I got interested in that space was there was a lot of talk in Silicon Valley about AI but most of that talk was hysterical in the sense that it was a form of magical thinking. You talked about the magic bullet; AI is the next magic bullet. I cannot tell you how many conversations I have been in where people tell me that “AI will solve that”, and “that” was everything from decision making, to org charts that don’t function, to finding the next “fill-in-the-blank”, and I know some of you are smiling because you have had the consultants tell you that AI is the answer to whatever the question was you had. Problem number one with that is no one actually defines their terms. So if I say artificial intelligence, you will all nod sagely, and I’m willing to bet sitting inside every one of your heads is a different definition. Because AI at this point is a lot like innovation: we all know it; we all think it’s a good thing; none of us actually want to know what it means specifically.
‘So how do you define AI? Well you have to do it in a couple of different ways. First you have to remember that this is an object that has a technical and a historic context. Artificial intelligence or the notion of machines that could think like humans has been around since the beginning of computing itself. The very first computers on the planet were described as electronic brains; the very first metaphors that we use to think about how computers worked were about the notion that they would think like humans could think. That gets crystallised in 1956 at a conference in America at Dartmouth College when a collection of mathematicians and philosophers lay out the very first artificial intelligence research agenda and coined the phrase. What they say back in 1956 is the challenge will be to minutely describe human activity, break it down into its sufficiently discrete component pieces that a machine can be made to simulate it. The notion was can you render all human activity into small enough pieces that we can describe it to a machine so a machine can do it.
‘In 1956, the things they thought that were most important for a machine to do was to understand human language—casual conversation not just structured conversation; [Secondly,] that the machine should be able to understand abstractions—so [understand] symbolic objects like a flag, not just recognise a picture of a cat (one of those is easy the other one is an abstraction). The third one was those machines should be able to learn over time. And the fourth one was that the machines should be able to reason, ie to be able to constitute an argument. Now in 1956 that was a pretty lofty goal, it took a very long time to get there, and I would argue we are still not altogether there. So the [term] artificial intelligence has a history.
‘If you were to look at where we are now in 2018 and define artificial intelligence I would argue that it’s five things plus one.
‘First thing you need to know when you’re talking about artificial intelligence is that it is impossible to understand as a single piece of technology; it is in fact a constellation of technologies. First thing you need to know is it involves data. You cannot have artificial intelligence without data. For those of you raised Catholic, data is artificial intelligence’s original sin. You will not get to AI without data but whatever that data is will shape AI profoundly and absolutely. So part one is a data set: you need to know where that data comes from, how it was collected, what the challenges are inside of its collection. Because there will be challenges even if they’re benign ones. I have colleagues in a scientific organisation in Australia who say their data [a collection of ocean images – Ed.] is biased and I asked them: “How?” They said: “Well it has a daylight and fair-weather bias”, and I said: “Why’s that?” “Because it was collected under water with cameras and you can’t throw someone off the back of a boat when it’s dark and stormy.” All data has a bias and you need to know what it is, because all artificial intelligence is built on a data set.
‘Second thing. All artificial intelligence contains a notion of an algorithm. Algorithms aren’t that complicated; they are simply a logical statement that automates a process. It says: “If A plus B then C.” All it says is a sequence of tasks—it’s all about can you shortcut something by making something do it automatically? You’ve all used an algorithm long before computers came along if you’ve ever used a washing machine, or had an automatic choke in your vehicle. All of those are algorithmically based; they just automate a series of processes. But inside artificial intelligence that same data set enables the machine to automate algorithms, so certain kinds of processes can happen without a human’s intervention.
‘Third thing. Inside AI technologies must be a notion of how the machine will learn, otherwise known as machine learning. There are lots of different techniques for them; some are just statistical modelling; some of them are slightly more opaque and complicated.
‘Fourth thing. Inside AI is the ability of the machine to sense the world around it so that it can gather new data in real time. That can be cameras. microphones, radar, GPS—any mechanism by which a piece of technology can know the world around it. Oh, and by the way, all the sensing that sits behind that.
‘Fifth and final thing is some kind of logical proposition for why that data is being collected, why the learning is happening, why the world is sensing things and why things are being automated. So what is the reason behind it or the strategy or the logic? I said it was five plus one because increasingly you can’t have a conversation about artificial intelligence without also talking about ethics, ie without talking about the broader context in which this is happening… what are the ethical constraints or the moral dimensions?
‘You can see why people say AI because otherwise saying data, algorithms, machine learning, sensing, strategy feels like a mouthful. But here’s the thing: AI is the beginning of a transformation, not the end; it is effectively the steam engine for a railway we haven’t built yet.
‘The World Economic Forum two years ago laid out the last 250 years of world history saying there [have] been three waves of industrialisation and we are now are entering the fourth one. We all know these earlier ones; mechanisation, electrification, computerisation. The last one is described by the World Economic Forum as cyber-physical systems but you might also think of it as the age of intelligence. Now the challenge with each one of these waves is that they not only produced technologies, they also produced significant social and cultural changes, they produced new laws, and they produced new threats and new possibilities in terms of both how you might produce military hardware but what you might do at scale, too. War changed with mass production and computers, we know it did. The notion of both the threat and the possibilities were there.
‘Of course what each one of these waves also did was generate the need for a new set of skills. The first wave brought us engineering. Then the second wave brought us electrical engineering. The third wave brought us computer science. It is hard to know what will come with that fourth wave but what you should think is that cyber-physical systems is a label under which is contained an entire class of machinery driven by artificial intelligence. And here I mean AI that gets off a computer screen and starts to find itself in physical things. The first classes of those would be autonomous vehicles also robots, but also includes banal things like smart elevators and smart buildings. It is anything that combines artificial intelligent technologies and a physical object. Those physical objects have the power to move and do things [in the physical world].
‘My challenge with this system is that we don’t yet know who the practitioners are that are going to help us navigate that fourth wave. I don’t know what we call them and I don’t know where to find them.
‘But I do know they have five questions they need to answer. First question is will those things actually be autonomous? That’s always been the promise that they’ll be autonomous and able to act without humans. But the question is what do we mean when we say autonomy in English? It’s a messy word; there’s a lot of semantic slippage. I say autonomy in English you think: sentient, self-aware, achieves consciousness; “Skynet” goes live and we all die. It is pretty much what happens. You get from autonomy to ‘Kill Sarah Connor’ very quickly.
‘Do we mean that it is fully an empowered actor on its own? Does it have a set of limits? Who is defining those? Who is granting the object autonomy and under what context? And frankly those things mean things differently in different culture systems, inside different regulatory systems and even inside different notions about what it would mean to be an autonomous actor. Sitting inside the room are people who all come from systems of governance and structure and I’m willing to bet sitting inside every single one of your founding legal documents there are different notions about what it means to be an autonomous human being; what it means to be a citizen is different from country to country. What the rights and responsibilities are—same problem here.
‘So let’s just take autonomous vehicles as the example: do we now mean that those vehicles are operating without ever having a human in the loop? Probably not. Do they sometimes have to check in with humans and under what circumstances? Are they autonomous the way our children under the age of 18 are autonomous? Like you need to know where they are at midnight, they’ll need to check in, and by the way you’re not going to let them have the new car. So they’re autonomous but limited. Or are they like your 20-year-old children where you worry about them completely differently? So when we say autonomy how are we actually structuring that? Because it turns out that has enormous implications for how you build it technically, how you secure it, what data flows through its networks, because if it is single-acting all by itself and it never needs to check in you’re going to need to pre-load it with a very different set of software and world views than if it is checking in once a day which is, for instance, the model for Tesla. All Tesla cars check in with the mothership once a day and they get little updates. You know there are different models of autonomy but answering that question is hugely important as we think about this entire class of new technical objects.
‘Second question: If an object is autonomous what is the nature of its agency, as in how much can it act without referring back to a rule set or without a human in the loop?This is complicated because rule sets are two things usually: rule sets can be explicit—the ones we know that we write down; and then they can be tacit—the ones that we use but don’t discuss.
Imagine you have a machine that may need to navigate both of those—it’s a little tricky. I’m willing to bet that what our friends in the Air Force think a machine should know may be different than what our friends on the land think a machine should know, which may be different to what our friends on the water think a machine should know. And by the way, it’s going to need to handoff between those forces and that’s a little bit tricky.
‘In Australia, in the state of Victoria, there is a very particular thing you do in a vehicle in the CBD, so in the central business district. It is called a hook turn; it probably goes against all the rules of nature. It involves taking a right-hand turn across multiple lanes of traffic in a manner that seems particularly silly. Imagine now we have an autonomous vehicle that needs to be taught to operate in Australia. We have granted autonomy and now give it Victorian rules and New South Wales rules. It will know how to do a hook turn in Melbourne but we will need to make sure it doesn’t do one in Sydney or Adelaide or Wagga because that would be bad. Now imagine that car came from somewhere else. It might have come from America. It will never know how to do a hook turn because you can’t teach it to do one because it’s irrational. So now we have a problem of an object that believes it should do one thing and needs to do something else. Imagine how it is that we’re going to determine what those rules are, who gets to set them, who gets to update them, how often they have to be updated, how they are transmitted, how they are negotiated and how they are scrutinised. Because all of those questions now sit here inside your robotic objects, in your autonomous vehicles. Whose rules? Oh and by the way in some of these instances you won’t want those rules sitting inside the object you want them sitting on the network on which the object runs.
‘It’s easy to imagine a moment in time we need all vehicles to come off the road. In Australia that might be an emergency services request because we have a fire you need to get everyone off the road so you can put a fire truck through a crowded area. So now every vehicle can be dismantled or disengaged from an external source.Who gets to decide that? How is that deployed? How is that enacted? [These questions] are all part and parcel of what this world would look like.
‘Which gets you to the third set of questions, which are about assurance, by which I mean safety, security, trust, risk liability, privacy, explicability and manageability. It’s a very long list. Also ethics sits under here, too. So now imagine you have that same autonomous vehicle. How do you know it’s safe? How do we determine what’s safe? How are we deciding what risks we are willing to endure with that object? Who gets to decide it?
‘Again, are those things innate to the object or do they sit outside of it? How are we imagining how that object will explain its actions? Because this is one of the things my colleagues in computer science won’t tell you terribly often, but a whole class of machine learning activities—basically ways that you can teach a machine to learn, particularly deep learning, what is otherwise known as unsupervised learning— where you ask a computational object to roam across a set of data and find patterns. The thing about that particular learning technique is that whilst it is powerful, the computational object cannot explain how it got to the conclusion it got to.
‘So it’s learned a pattern in the data that it cannot tell you about and it is now acting on that pattern. Pause for a minute and think about what that would look like in a highly regulated environment or one that is subject to post action scrutiny. So do you want an object that has acted but cannot explain why it did that? I’m willing to bet we don’t want that in certain sectors, yours may well be one of them. So what it means to have what is called back-traceability, or the ability of an algorithm to explain why certain actions happened, is actually technically quite tricky and at the moment may be a cause, under certain circumstances, to say of robotic objects [that] you don’t want certain classes of machine learning techniques enacted. So in fact you may need to be putting limits on how these systems work because they can’t explain their actions afterwards, which would create different problems.
‘[The] fourth set of questions here I think that are necessary to answer in this new world are about metrics, so how we know if these are “good or bad” systems, crassly put. Over the last 250 years most new machinery has been understood by either increases in productivity or increases in efficiency. I’m not sure those are the metrics that we want to use here. Ought to it be about safety? Is it about not having humans in the last 300 meters, and not having wet socks and being in the mud? Maybe that’s a different metric. Having a machine replace a human; the metric there is about human life. That’s not necessarily an efficiency call.
‘It’s also the case again that there are a number of pieces [of computations] that sit inside artificial intelligence that are actually incredibly energy intensive. So if you are sustaining a set of technologies far from reliable and robust infrastructure there are certain kinds of techniques that you may not want to use. In mid-2018 the last statistic I read was that about 10% of the world’s energy budget is now being spent on server farms. That’s a big number and it’s only going to get bigger. So now imagine you are attempting to negotiate a deployment of cyber-physical systems on a battlefield. How are you going to power them if they choose to use certain kinds of computational techniques that are energy intensive? Deep learning is the most energy intensive set of algorhythmic workloads that I know of. Imagine running deep learning off a truck battery or even a generator; [it’s] a little hard to comtemplate. How we might build these systems with sustainable metrics in mind, and energy sensitive ones, is a whole new way of thinking about these systems and not something anyone is thinking about currently.
‘And last but by no means least how is it that we imagine we’re going to engage with these systems? I think that was your last question, Kath, and it’s a good one. At the moment we engage with computing in a very narrow way; it’s a keyboard; it’s glass; sometimes it’s voice; very occasionally it is gesture. We are now talking about systems that don’t require humans to act. We are talking about systems that we may find ourselves inside; we may find ourselves with systems negotiating around us. How these systems will signal what they are becomes hugely important.
‘I was joking earlier about teenage kids and cars; we know how to signal vehicles when we have teenage children in them. They have an L plate which says don’t get behind them when they are reverse parallel parking. We all know how to read that as a sign, right? How do these systems get signal to us? How do we know what they are? How will we engage with them? How do we not bring the metaphors of the last 100 years of computing with us so that we are not putting the moral equivalent of yet another little square disk for saving? We don’t even know what that means anymore, but the icon to save something on your computer is, in fact, a three [sic] by five disk. Most of us in the room are old enough to remember that; many are not.
‘Imagine what these systems will be like if they had to require passwords; if you had to talk to them. All of those are not extensible so how we think about how these systems will work with one another is actually complicated. This leads me to two final points; one, if the world we’re moving to is one of cyber-physical systems, where robots are just the first class; autonomous vehicles are just another piece of a broader puzzle, all of those questions are complicated. They have huge implications for how you think about everything, including really boring things. For example, how are you going to write a tender for a cyber-physical object?
‘Take the Hawkei; how are you going to write the tender for the next one of those? It is going to have a strategy engine in it. On whose data has it been trained? Whose strategic intent is it enacting? Do we want to have someone else’s strategic intent inside our computational object and if not how do we determine that? How are we going to train people to manage those systems? Start from the tender and work your way forward. How do we train a generation of people to work with these objects? How do we train the people who are going to repair them? How do we think about their battle needs—if that’s where you want go— that have to do with power and network needs and security needs?
‘These are not just opportunities they are incredible vulnerabilities and thinking about how they are secured and built and managed isn’t just as simple as saying: “They’re emerging technologies, AI is the answer”. What you actually have to think about is what is the question you are trying to answer and why, and what will the pitfalls and perils of all of that be?
So with that I’m going to stop and say thank you.’
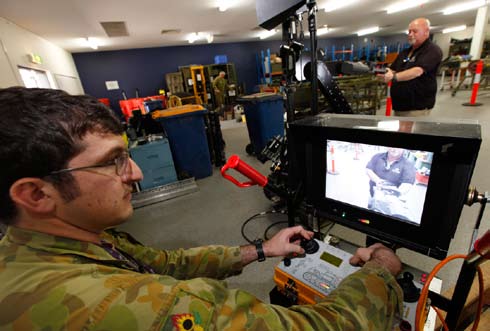
Figure 36. A robotic explosive detector is tested at the Regional Explosive Ordnance Service. (Image: DoD)
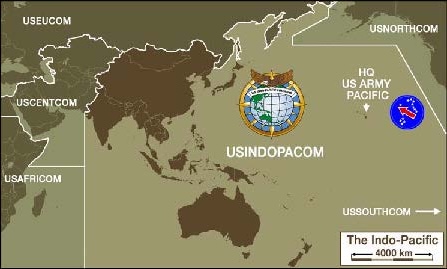
Figure 37. The US recently renamed its functional command area from USPACOM (US Pacific Command) to USINDOPACOM (US Indo- Pacific Command) signifying the importance the region plays to Australia’s primary ally. It is interesting to note that it is the only command that shares borders with all the other functional commands. (Image by Major Conway Bown)